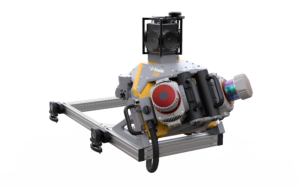
With Trimble’s next generation mobile mapping system, all sensors are time synchronized with precise GPS time tags and are linked to the trajectory that is recorded with the GNSS/IMU subsystem. That way, all recorded points and images can be properly aligned in a post-processing step,
The steady climb up the slope of improving sensor technology is generating better geospatial solutions, especially in mobile mapping and machine control, and as a result, improving workflows, productivity and project outcomes. What is most exciting about data collection right now is the potential to bring together multiple different sensor types or technologies to advance geospatial solutions in the areas of cost, performance, availability and reliability.
Editor’s note: A PDF of this article as it appeared in the magazine is available HERE.
For users of LiDAR and other singlesensor collection technologies, the combinations of data from different types of sensors into one solution can generate more useful data from missions, which can improve productivity and expand services.
In this way, what is most exciting also poses the greatest challenges for engineers and product developers who need to create hardware and software solutions that integrate multiple types of data to generate results users can rely on.
While not necessarily something users are demanding (yet), this sensor fusion is one of the leading edges of product development because of the power that comes from combining multiple different sensor types or technologies in ways that maximize their combined strengths while minimizing their combined weaknesses.
Under this backdrop, it’s helpful to consider the technology and market trends driving the convergence of data from various and different types of sensors–whether inertial measurement unit (IMU), camera, barometer, Global Navigation Satellite System (GNSS), or Light Detection and Ranging (LiDAR)– and the software that will process the data from those disparate sources to deliver a continuous and unified stream of information.
Market motivation
For the geospatial market, there are multiple goals in maximizing the benefits of sensor fusion, including reducing costs, improving performance to get better positions or availability of positions, and increasing reliability and robustness. Geospatial technologists are finding new ways to fuse the data from diverse sensors in an effort to make positioning with centimeter accuracy possible in any location and environment, such as enabling a GNSS user to walk indoors and maintain a high level of accuracy in data collection or walk into a forested area and maintain sub-meter or centimeter accuracy despite interruptions in line of sight to satellites.
Increased computing power and advancements in GNSS have improved the performance of geospatial solutions, but to continue to get better, technology needs to make greater use of existing sensors and also incorporate additional sensors for new sources of data.
As one example, consider that when data generated from an IMU (inertial measurement unit) and GNSS are integrated, the system gains the dependability and consistent accuracy of GNSS with the continuous data propagation of the IMU, which is useful in environments such as an urban canyon where GNSS signals can be interrupted.
Autonomous vehicle technology sparks sensor innovation
The autonomous vehicle push has brought additional attention to geospatial solutions because of the need for multiple sensors beyond GNSS, including LiDAR and cameras. A driverless car in the same environment as vehicles operated by drivers can’t rely on GNSS alone because that system won’t work in certain conditions, such as tunnels, and can’t pick up unexpected hazards, such as pedestrians walking on the street.
To work at all times, the driverless system needs multiple sensors. Improved vision and depth-sensing solutions are among the leading-edge technologies being used in the autonomous vehicle sector to compliment GNSS.
Inertial sensor technology gets smaller, better, less costly
At the same time, some sensors, such as the IMU, are now getting smaller and much less expensive.
The IMU is an electronic device that uses a combination of sensors–accelerometers, gyroscopes and sometimes magnetometers. An IMU measures specific force in three directions (forward/backward, left/right and up/ down), as well as angular rate on three different axes. These measurements can be used to compute the body translation and orientation change over time.
Until fairly recently, inertial systems have been expensive, large, power-consuming and heavy, qualities that made them acceptable for a train or an airplane but a poor choice for smaller, more typical applications, like vehicles.
Inertial sensors measure specific force (acceleration excluding gravitation, so zero during free-fall) and angular rate. These measurements indicate motion changes. This movement in space is interesting in some applications, but geospatial users want to know where things are, not just where they are relative to other things. Another strength of the IMU is high measurement rate, typically 50 to 2,000 times per second.
Recent improvements in IMUs, and the advancement of Micro Electrical Mechanical Systems (MEMS) in particular, have made the inertial systems smaller and more cost effective. One example is the smart phone, which includes tiny inertial sensors that cost only a few dollars. These sensors aren’t quite the quality needed for geospatial solutions, but they are getting close.
When inertial sensors are combined with GNSS, the system is able to estimate both position and orientation, which are important for guidance and navigation.
While GNSS needs to be able to see the sky and the satellites, an IMU does not. The weakness of the IMU method is that it is not stable over time, so its ability to determine position drifts very quickly with time. GPS is almost the opposite. In the short term, GPS has a slow uptake rate, but over the long term, it doesn’t drift.
Vision sensors create rich data
On its own, a camera is a rich source of information. But that data is also a disadvantage because of the large amount of processing it requires, and state-of-the-art systems are still not 100% reliable due to variable lighting conditions. For geospatial purposes, the camera needs to be integrated with a sensor that provides absolute scale, like GPS, inertial, laser, or additional cameras.
Computer vision, or structure from motion, is an increasingly strong focus of geospatial technology. Computer vision uses a camera to take a photo or video and calculates movement and rotation based on changes in consecutive frames. The problem with computer vision is that the scale isn’t known, similar to how the size of a tree can’t be determined from a photograph of that tree because the distance between the camera and tree is unknown.
On its own, computer vision can provide a model of the scene to an unknown scale, but a geospatial use requires integrating the vision sensors with GNSS or inertial to calculate absolute positions and position changes.
Mobile mapping combines sensors in powerful way
Mobile mapping systems exemplify the power of sensor data fusion by combining the various strengths and weaknesses of different types of sensors–inertial (IMU), wheel speed, GNSS, cameras and LiDAR–and fusing these sensor outputs into one large data set with many uses.
While a stand-alone, single-sensor solution will cost less than a multiple-sensor solution, the increased productivity gained from multiple sensors saves not only money, but time. Mobile mapping solutions allow efficient data acquisition over large areas from 10s to 100s of kilometers at highway speeds. In environments where safety might be an issue, such as an unclosed road, surveygrade data can be acquired without endangering ground crews. Such a system is particularly useful in transportation infrastructure planning, as-built surveying, GIS mapping and asset management.
Trimble’s new MX9 mobile mapping system is one example. The MX9 combines multiple sensors, including two super-accurate high-density lasers that collect one million points per second, per laser, to generate survey-grade dense point cloud data. The lightweight system also combines a high-end Applanix GNSS IMU component and a spherical camera to get great images. All sensors are time synchronized with precise GPS time tags and are linked to the trajectory that is recorded with the GNSS/IMU subsystem. This way, all recorded points and images can be properly aligned in a post-processing step.
Processing sensor data
Sensor fusion is also about getting the most out of one data acquisition mission, and the more information collected, the greater the need for a smart system to handle it and avoid a data graveyard.
From a user’s perspective, gathering data using a mobile mapping system is fairly straightforward, but once the data is collected users face the challenge of processing and analyzing the information.
The processing step is where the sensor fusion is needed. When GNSS is available it is an excellent source of absolute position, but because it requires line of sight to the satellites, there are many times when the positions are either degraded or completely unavailable due to obstructions. It also does not generally provide user orientation, which is required for mobile mapping. During these outages, sensor fusion becomes a requirement. By combining inertial, camera, LiDAR and odometer data, it is possible to maintain position and orientation during these inevitable outages. The quality of the fused solution depends on many factors, including the duration of the outage and the distance traveled.
Analysis of the data is the process of extracting information of interest. This generally involves detection and measurement and can range from fully automated analysis to a fully manual process. Common examples include detection and measurement of street furniture, road surface defects and curb mapping.
Having different types of sensor data can be extremely powerful, but even more beneficial is fusing that data into one analysis. The right software is critical to calibrate and co-register the data properly so it is presented in an accurate way. This means fusing the different sensor data sets, such as combining the individual points, or non-continuous data, from LiDAR, with the continuous data of edges and lines from cameras.
Supported by the right software, sensor fusion is about getting the most out of various sensors and sensor combinations to solve business problems.