Introduction
3D city models have found a wide range of applications such as smart cities, autonomous navigation, urban planning, and mapping. However, existing datasets for 3D modeling mainly focus on common objects such as furniture or cars. Current urban modeling datasets have been restricted to small datasets [1] or synthetic ones [2]. Lack of building datasets has become a major obstacle for applying deep learning to specific domains such as urban modeling.
The Building3D datasets consist of more than 160,000 buildings along with corresponding point clouds, meshes, and wireframe models, covering 16 cities in the Republic of Estonia, a total of about 998 km2, as shown in Figure 1(b and c), 1d and 1e, respectively. The goal of Building3D is to provide state-of-the-art data sets to research communities for advancing urban modeling research in photogrammetry, computer vision and remote sensing.
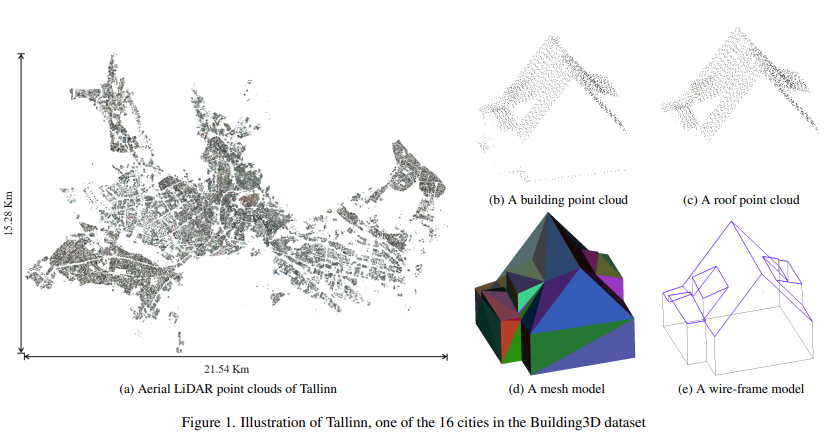
Figure 1: Tallinn city and its corresponding building and roof point clouds, meshes, and wireframe models in Building3D
Dataset analysis
We process the raw data provided by the land board of Estonia to generate the Building3D dataset (Building 3D model data: Estonian Land Board 2022). Figure 2 shows overall statistics of the proposed dataset, which contains about 160,000 building point clouds with corresponding mesh and wireframe models. Figure 2(b) shows histograms of point clouds and objects (i.e., buildings) in each city. The orders of magnitude of points and objects are indicated by symbols M (million) and K (thousand) respectively. Figure 2(c) shows the area of each city. Figure 2(d) shows the average memory consumption of a building point cloud, mesh, and wireframe model in each city respectively. The average memory consumption of a building point cloud is between 83.89 and 672.97 KB, while that of the corresponding mesh and wireframe model is less than 6.5 KB and 2 KB respectively. The ratio of average sizes among building point clouds, mesh and wireframe models is approximately 400:4:1.
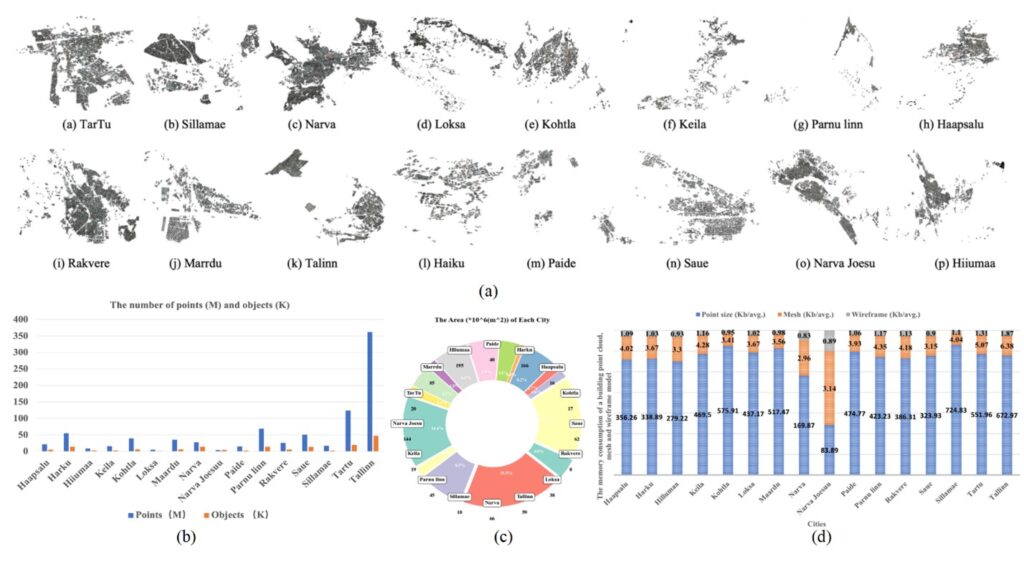
Figure 2: Details of Building3D dataset. (a) Illustration of 16 cities in our dataset. (b) The number of points and objects in each city. (c) The area of each city. (d) The average memory consumption of a building point cloud, mesh and wireframe model in each city.
Buildings analysis
We anticipate that Building3D and its corresponding benchmarks will bring forth new challenges and opportunities for research and industrial applications.
The dataset presents three primary advantages:
- Authentic real-world representation: Distinguished from existing artificially constructed building datasets, Building3D comprises buildings from Estonia, reflecting real-world structures. As depicted in Table 1, we computed the minimum, maximum, and average number of corners and edges in the roof structures. The results indicate that Building3D exhibits higher complexity compared to existing data.
Dataset | Minimum | Maximum | Average |
Corners // Edges | Corners // Edges | Corners // Edges | |
Synthetic dataset | 4 // 4 | 11 // 15 | 8 // 10.6 |
Bulding3D | 4 // 4 | 52 // 65 | 15.7 // 32.8 |
Table 1: Comparison of corners and edges between synthetic and Building3D.
- Diverse range of categories: Building3D includes a vast collection of around 160,000 3D point cloud buildings encompassing over 100 distinct roof types (Figure 3).

Figure 3: Illustration of diverse roof types in Building3D.
- Abundant annotations: The dataset includes point clouds, wireframe, and mesh models of roofs as illustrated in Figure 4.
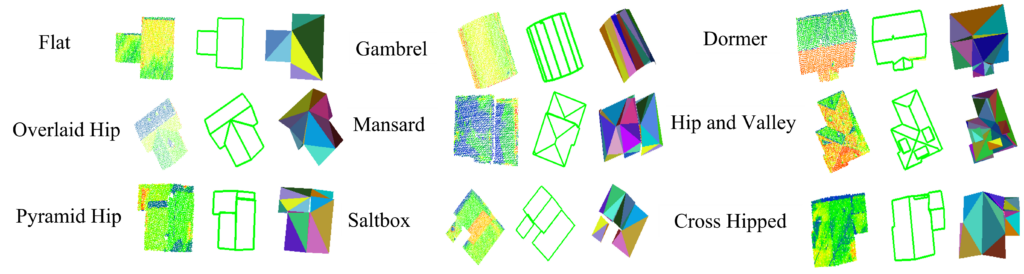
Figure 4: Illustration of diverse roof types in the Building3D.
Downstream task
Building3D has provided significant research prospects within the research communities. In addition, we also proposed a unified, supervised, and self-supervised end-to-end framework for 3D building modeling, dividing the reconstruction task into two primary components: 1) detecting and recognizing building edges and corners, and 2) establishing effective edge connections among buildings. This dataset can be employed for the evaluation and analysis of the 3D building reconstruction.
Conclusions and future work
In this paper, we present an urban-scale dataset for building roof modeling from aerial LiDAR point clouds. Besides mesh models and real-world LiDAR point clouds, it is the first time to release wireframe models which transforms 3D building reconstruction into a classification problem. We believe that this work will help advance future research on several fundamental problems as well as common object modeling such as mesh simplification and remeshing. In the future work, our commitment lies in its continuous expansion and updates to cater to diverse research needs. Beyond its current applications, we are actively planning to explore additional downstream tasks, including sparse point cloud completion and semantic segmentation etc. In addition, we aim to add detailed building facade models to enable LoD3 modeling for photorealistic building model generation, and associate address data to each building for holistic 3D scene understanding.
Note about this article
This is a slightly shortened version of a published paper by the same authors, which is listed as the third reference in the bibliography below. The Building3D dataset released by the spatial intelligence lab at the University of Calgary is the first and largest urban-scale benchmark dataset for 3D building reconstruction from aerial point clouds.
Project website: http://building3d.ucalgary.ca/
Paper URL: https://arxiv.org/pdf/2307.11914.pdf
Bibliography
[1] Wichmann, A. et al., 2019 RoofN3D: A database for 3D building reconstruction with deep learning, Photogrammetric Engineering & Remote Sensing, 85(6): 435-443.
[2] Li, L. et al., 2022. Point2Roof: End-to-end 3D building roof modeling from airborne lidar point clouds, ISPRS Journal of Photogrammetry and Remote Sensing, 193: 17-28.
[3] Wang, R., S. Huang and H. Yang, 2023. Building3D: An urban-scale dataset and benchmarks for learning roof structures from point clouds, International Conference on Computer Vision (ICCV 2023), Paris, France, October 2 – 6, 2023.
___
Dr. Ruisheng Wang is a professor in the Department of Geomatics Engineering at the University of Calgary (UCalgary), which he joined in 2012. Before that, he worked as an industrial researcher at HERE Technologies (formerly NAVTEQ) in Chicago, since 2008. His primary research focus there was mobile lidar data processing for next-generation map making and navigation. Dr. Wang holds a Ph.D. in electrical and computer engineering from McGill University, an M.Sc.E. in geomatics engineering from the University of New Brunswick, and a B.Eng. in photogrammetry and remote sensing from Wuhan University.
Shangfeng Huang is currently working on his PhD. As a member of the spatial intelligence lab at UCalgary, his main research interests focus on 3D building reconstruction from point clouds.
Hongxin Yang is also currently working on his PhD and is a member of the spatial intelligence lab at UCalgary with research interests in 3D building reconstruction from point clouds.