Using mobile lidar for Roadway Characteristics Inventory (RCI) projects seems ill-advised. On deeper examination, however, it’s a viable solution. Based on the name alone, some may think that RCI is just Building Information Modeling (BIM) for roadways, and that is exactly what it is. Traditionally, RCI projects have been linear-based referencing systems using starting and ending points, such as mileposts or intersection-to-intersection, to identify features and their positions relative to the start and end points.
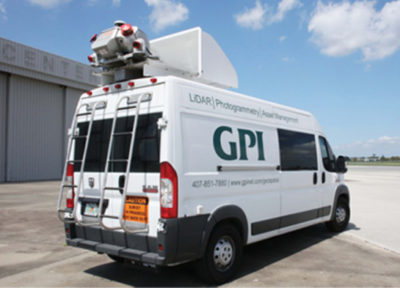
GPI’s mobile van, used for data collection on the pilot. The RIEGL VMS-450 lidar system can be seen mounted on the roof.
Several different tools are used to capture the required information to create and properly attribute features. These can range from a video log to “boots on the ground” assessments. Since we are already mobilizing to the project site driving video, why not use mobile lidar that has GNSS, lidar, imagery, IMU, and georeferencing video capacities? Being able to convert from a linear system to a GIS management system justifies looking into the possibilities of what can be achieved with a geographically-based RCI system.
Florida Department of Transportation (FDOT) and many other clients have been asking similar questions. FDOT recently contracted GPI Geospatial, Inc. (GPI) to perform an RCI pilot project. Before describing the specifics of the pilot, however, we discuss some of GPI’s capabilities that gave the confidence to take on an RCI pilot project. GPI owns and operates two RIEGL VMX-450 dual-channel lidar sensors. Some may wonder why we don’t use the VMX-2HA, but we consider the VMX-450 to be the most hardened of the VMX systems. It is relatively low noise and very dependable. Although the VMX-450 suffers transfer speeds that limit still imagery capture rates, GPI has added a georeferenced video component, which raises the bar.
GPI has performed a wide variety of projects for FDOT, other municipalities, and private developers that helped to qualify us for this opportunity. For many years, GPI has performed survey-grade mapping using mobile lidar for engineering design all over the eastern seaboard and across to Minnesota. GPI has not only used mobile lidar on these projects but has developed streamlined workflows to acquire and merge aerial lidar, static lidar, and traditional surveying to make a single seamless survey-grade base map for the use of engineering and design projects. We also provide uncontrolled GIS work.
One of GPI’s largest markets was sign inventory: we collected data and built GIS databases for the Massachusetts Department of Transportation and the New York City Department of Transportation. More recently, GPI provided lidar and imagery of several remote roadways to the Maine Department of Transportation for roadway conditions assessments. GPI is also integrating a georeferenced video system into our mobile lidar—this is still in the early stages of development but functional. We are expecting our video capabilities to grow substantially over the next year.
The FDOT pilot consisted of approximately 28 centerline miles of mobile lidar data acquisition and processing to determine whether mobile lidar could be used to replace traditional RCI collection methodologies for a statewide program. There were many unknowns for both the contractor and the client, of which the biggest was relative and positional accuracy. Once the accuracy component was proven, the rest of the project was simply data delivery and data extraction. The RCI’s linear referencing model had adopted an acceptable relative accuracy requirement, so to justify a complete overhaul of the RCI system, GPI had to push the limits of both relative and positional accuracy.
FDOT and GPI decided to set both accuracy thresholds at ±0.25 feet (±0.076 m). Most lidar system suppliers claim the absolute accuracy of untargeted mobile data is about ±3.28 feet (±1 m). For GPI to achieve the accuracies required we relied on our extensive experience in surveying, geomatics, and extensive mission planning. We had a consistent outlook on the project as a scalable statewide mapping project instead of just focusing on the 28 centerline miles of the pilot project.
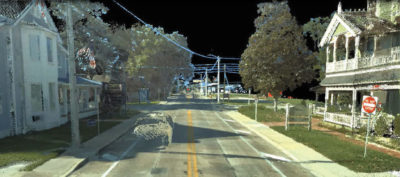
Samples of data processed by GPI. Above: colorized point cloud with street-level perspective; Below: rail road crossing feature fitted to colorized point cloud, imagery captured by van, and intensity point cloud.
One of our biggest concerns was the method of applying the corrections to the mobile GNSS observations. We briefly discussed the use of RTK via modem to receive corrections, but that idea was discarded owing to the unreliability of statewide cell coverage. Instead, we decided to post-process our GNSS/IMU data using POSPac. Another concern was baseline and mission drift. GPI requested that FDOT allow us to test out the Florida Permanent Reference Network (FPRN) according to several different plans. The first was to use a physical FPRN base station. This was a “tried-and-true” processing method but, like RTK correction, lacked coverage. The second plan was to use the virtual base station derived from the FPRN. This solved the coverage issue affecting the first plan, but did the virtual base points have the repeatability and reliability that a physical base station could provide? The third plan was to use a network of virtual base points surrounding the mission area, so as we moved away from the pilot area, we could use at least one common virtual base station between missions. This plan provided coverage reliability and scalability.
The first plan was chosen to perform the downstream task of the pilot. The first and second plans had remarkably equivalent results, which reassured us when using the virtual base points derived from FPRN. The first plan had a slightly better fix/float ratio to the mobile GNSS/IMU observations but we would feel comfortable using either approach. Unfortunately, the third plan gave inconclusive results. POSPac’s smart network tool did not accept the virtual base data. We are continuing to pursue this issue with Applanix with a view to future projects.
Using our best GNSS approach we processed the lidar and imagery. FDOT placed ground control but we did not receive the locations or values, so the pilot was a blind accuracy test. We began analyzing the data by comparing adjacent passes, like comparing traditional GNSS RTK observations to identify the error between passes. In general, the average of multiple observations is most likely the true value. With that in mind, we moved forward to QC/QA checks. As expected in areas with few GNSS obstructions, the error was low with discrepancies of about 0.15 feet or less between passes. In areas with GNSS obstructions, such as building canyons and tree lines, the error jumped up to 0.3 feet between passes. When the discrepancies between adjacent scans were around half of the agreed error of ±0.25 feet, GPI had confidence that we met blind test expectations of the pilot. We decided to move forward and delivered the raw, uncalibrated lidar, imagery, and video.
Since GPI always tries to push limits and increase expectations, another component we asked to incorporate in the pilot was a limited relative calibration of the raw data. We have developed a unique approach for calibration using the RIEGL software. It allows us to focus on areas that are out of tolerance around route corridor crossings, so we can provide limited calibration using a weighted average instead of the typical options of full calibration or raw data delivery. We explored this option with the intent of reducing the cost of full calibration when scaled statewide. GPI wanted to provide an additional level of quality at acceptable cost.
This took our data from the raw 0.15 feet of error to an average of 0.05 feet between passes in most areas with limited GNSS obstructions. Even the 0.3 feet discrepancies were reduced to 0.05 feet of error between passes. Although this adds cost upfront, the improved quality should allow a technician to load multiple passes to decrease voiding from moving traffic and increase point density when looking for features, which will increase efficiency.
With the data acquired, it is essential to understand what can be done with mobile lidar and associated imagery. In general, lidar allows more experienced people the opportunity “to be a rodman again”. This simply means it is possible to extract exactly what is required for the project. This process eliminates the need for work to be repeated, which increases the cost. On top of increased productivity, the level of data mining is almost limitless. In addition to the obvious features, such as signs, striping, and other pavement markings, it is possible assess features such as Americans with Disabilities Act compliance, roadway condition, drainage flow, etc., from the lidar point cloud. In addition to what can be identified, extracted and assessed with just lidar, the georeferenced imagery, colorized point cloud, and video are available to assist further in the data mining process.
Like everything else in our industry, processes are rapidly changing and improving. If its methods do not change, a firm can quickly become obslolete. Converting RCI from a linear reference system to a graphical system allows the client to know not only what assets are there but also where they are. Adding mobile lidar allows for precise location and assessment of features without “boots on the ground”, while allowing planning and development studies with the data. Additionally, GPI has been developing its tools to include high-resolution georeferenced video, oblique stereoscopic imagery, and artificial intellegence capabilities. Thus the answer to the question, “Is it possible to use mobile lidar for RCI projects?” is emphatically positive. Firms and their clients who have not begun to use mobile lidar for RCI are missing a major opportunity.
Trevor Tyson has 15 years of experience in surveying and lidar services. His duties have ranged from rodman to leading all aspects of the mobile lidar department at GPI Geospatial, Inc. In addition to his traditional survey expertise, his lidar experience includes mission planning, acquisition, GNSS/IMU processing, calibration, extraction, and creation of final deliverables utilizing aerial, mobile, and static platforms. Trevor is passionate about geospatial services and all the unexplored possibilities in this industry.