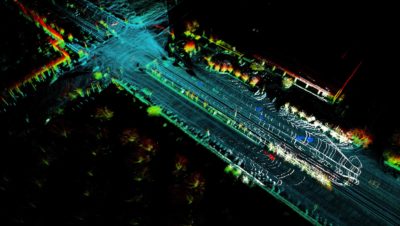
Figure 1: The white points from a single frame of lidar data are shown in the context of a geolocated map. The registration of real-time data against a prior map is a powerful tool for localization.
Perception for any robotics task involves both the inference and prediction of reality from a set of imperfect sensors. All sensors seek to measure that reality to inform perception. Cameras, for example, produce densely sampled data in angular coordinates. At the individual pixel level, however, the data is nearly meaningless, so computer vision algorithms operate on patches of pixels to accumulate meaning. Radar provides a different slice of reality by directly measuring range and velocity, but with very coarse angular resolution. To complete this puzzle, lidar’s three-dimensional acuity is widely recognized as indispensable for safe autonomous navigation. Localization against pre-existing maps is a standard use-case for lidar data (Figure 1). However, due to its limited range, lidar has not been more widely adopted for broader perception tasks such as object detection and tracking. The unique advantages of FM lidar for object detection and tracking is poised to expand how lidar is utilized.
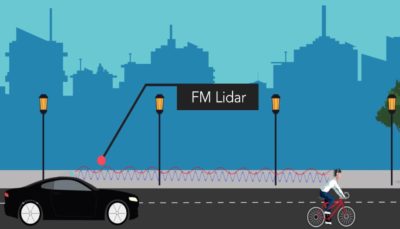
Figure 2: An FM lidar transmits continuous wave (CW) light to a target (red). The backscattered light (blue) may be Doppler-shifted depending on relative motion between the sensor and an object such as the cyclist. The shift is measured in a handful of microseconds and exists as a unique velocity measurement on every single point in the point cloud.
Frequency modulated, continuous wave (FMCW) lidar relies on coherent detection, or the detection of backscattered laser radiation, via the optical interference of that signal with a local copy of the optical source (Figure 2). Coherent detection offers both interference immunity (solar and lidar-to-lidar) and single-photon sensitivity. This combination enables operation to distances beyond 200 m within the optical power constraints of integrated photonics platforms. Perhaps the most compelling attribute of coherent detection is that Doppler shifts of the reflected signal can used as high fidelity estimates of radial velocity.
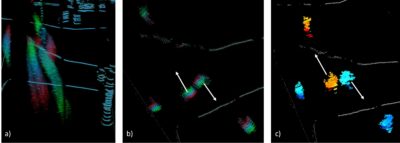
Figure 3: a) Data from Blackmore’s LDP lidar product. A set of points colored by ‘age’ demonstrate pedestrian tracks in an intersection. Red is older, green is newer. b) An overhead view of age-colored points shows two pedestrians passing. Velocity derivation of the pedestrians requires comparison of the points over time to infer motion. c) Doppler colored points of a single FM lidar frame clearly show pedestrian velocity on each data point. Furthermore, limbs and torso motion are delineated.
In the context of autonomous vehicle (AV) sensor packages, radial velocity measurement has hitherto been limited to radar platforms. Typical automotive radar carrier frequencies (24 or 77 GHz) lead to Doppler shifts in the kilohertz frequency range. To measure these frequency shifts, radar signals must be processed over tens of millisecond intervals to achieve adequate frequency resolution. While the range resolution of such radar signals can be a few centimeters, practical phased array apertures, even with MIMO (multiple-input and multiple-output) techniques, limit angular resolution.
FMCW lidar operating at 1550 nm is essentially a radar system operating at a 193 THz carrier frequency. This carrier frequency allows very high angular resolution—the laser beam divergence can be <0.01 degrees—as well as an extreme sensitivity to the Doppler effect. In fact, it’s possible to achieve velocity resolution comparable to automotive radar at processing intervals of only a few microseconds. The combination of these effects leads to a much higher resolution data product with much lower latency. The result is a high resolution, velocity-enhanced point cloud—a sort of ‘radar on steroids.’
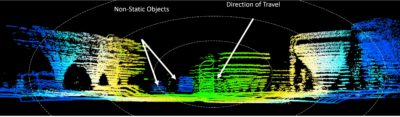
Figure 4: The color displayed in a single frame of Blackmore FM lidar data is determined by raw velocity. Green indicates the fastest approaching velocity with dark blue the slowest. The fastest relative velocities are shown in the center of the frame in the direction of travel. Away from the center, the relative velocity decreases as the projection of the true velocity on the beam direction. Some moving cars in the scene show different velocities. Such data sets can be fit to measure true global velocity independent of other sensors.
FM lidar improves the usefulness of conventional lidar data and streamlines the automation of general AV data annotation. By fusing velocity-enhanced lidar data with camera and radar data, moving objects are more accurately resolved at finer time scales and longer ranges. Offline, this reduces the need for manual annotation of data sets—an expensive and unscalable process that will fail to keep pace with (and extract value from) AV data produced by large fleet operations. For online perception, the fused data product has a multiplicative effect on signal confidence. Single-frame observations, poor visibility, or cluttered scenes all benefit from FM lidar. For example, when several pedestrians are crossing paths, it can be very challenging to predict their separate and distinct paths. FM lidar helps to maintain distinct object tracks, and prediction confidence in cluttered environments (Figure 3).
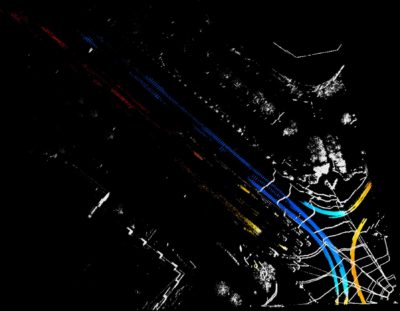
Figure 5: An overhead, Doppler-colored view of several seconds of data at a busy intersection in San Jose, California clearly shows traffic patterns. Cross-referencing this data greatly improves the ground truthing of radar sensors and streamlines the development of angle-of-arrival algorithms for such sensors.
In automotive scenarios, FM lidar point clouds include millions of independent measurements of velocity across the sensors’ field of view (Figure 4). These data are used to produce an accurate estimate of vehicle velocity that are independent of INS solutions, wheelspin readings, or localization routines. This is achieved with remarkable efficiency, and without requiring additional analysis such as frame-to-frame point association, feature extraction, or surface normal estimates. Velocity state estimation from FM lidar data improves SLAM routines and can also reduce INS hardware or high-definition map requirements. The same velocity estimate can help refine high-resolution mapping products and allow for extremely cost-effective removal of transient objects from the map data. Both online and offline, FM lidar addresses several localization challenges.
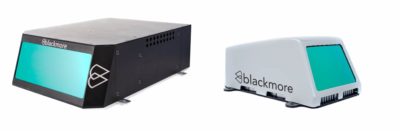
Figure 6: Blackmore’s Autonomous Fleet Doppler Lidar (left) and Lidar Development Platform (right) support customers in broader autonomy space with real-time, FMCW lidar. See blackmoreinc.com for more details.
Many have asked if lidar (especially FM lidar) will one day replace radar. Indeed, the velocity measurement of FM lidar is much lower latency than radar. Furthermore, the angular accuracy of FM lidar makes it an ideal reference sensor for the verification and testing of automotive radar detection and tracking algorithms (Figure 5). However, radar retains performance advantages in bad weather and sensor blockage. For personal vehicle safety, we don’t have to choose between an airbag and a seatbelt. For autonomous driving perception, FM lidar and radar provide the same complementary benefits; why would you choose just one?
About Blackmore
Blackmore Sensors and Analytics was founded in 2016 with the mission of commercializing FMCW lidar technology and associated data products. The founding team has worked together on coherent lidar, primarily for U.S. DoD applications, for over 10 years and has contributed several academic papers in the field of coherent imaging. Today, the Blackmore team consists of over 75 multidisciplinary engineers and scientists to cover the broad needs of well-developed lidar products (Figure 6). Blackmore is based in Bozeman, Montana.